Data science is a field that is at the forefront of innovation and insight in the data-driven world we live in today. Data science is becoming a key field that is influencing industries all over the world by utilizing the power of sophisticated computer tools, statistical approaches, and domain expertise.
This paper provides a thorough overview of the complex field of data science as a course, elucidating its complexities and emphasizing its pivotal role in contemporary society.
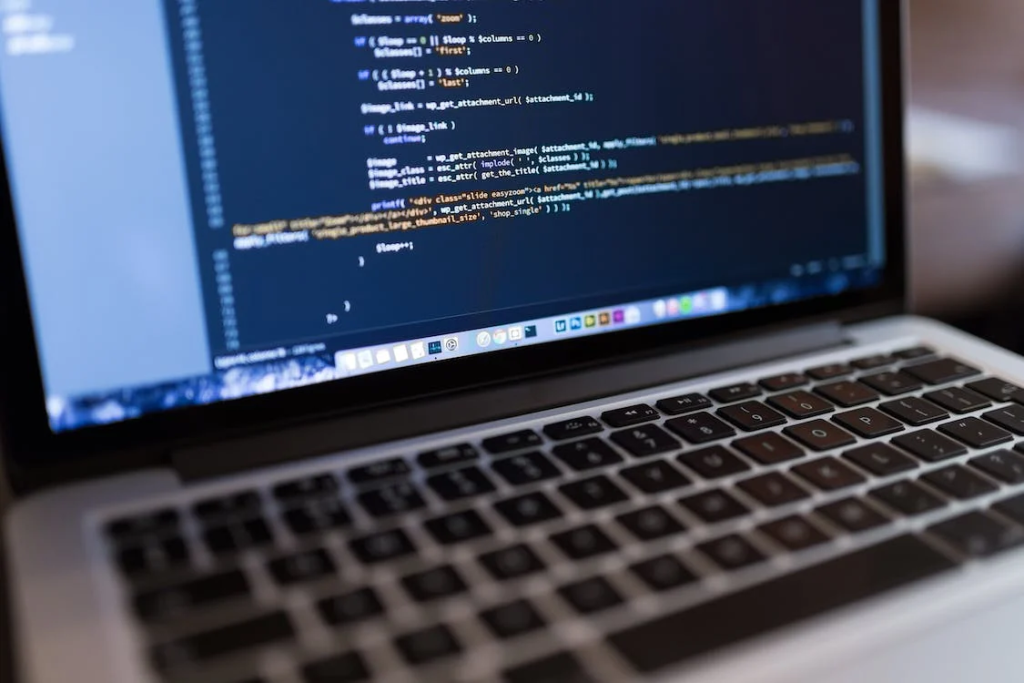
A comprehensive approach to comprehending and utilizing data for well-informed decision-making can be found in academic data science courses. A wide range of topics are usually covered in these courses, from cutting-edge methods in machine learning and artificial intelligence to basic ideas in mathematics and statistics.
Using a combination of academic knowledge and practical applications, students acquire the analytical skills necessary to derive significant insights from intricate datasets, thereby promoting innovation and propelling organizational success.
To put it simply, taking courses in data science opens the door to realizing the transformational power of data. These courses equip students to deal with the complexity of the modern world with confidence and creativity by providing them with a thorough understanding of data processing, visualization, and interpretation.
As we continue to explore the nuances of data science as a course, let’s go on an innovative, exploratory, and discovering trip to uncover the countless prospects that lie ahead in this fascinating profession.
What Is Data Science As A Course?
When we talk about a data science course, we usually mean an educational program or curriculum that is provided by specialist training facilities, online platforms, or academic institutions.
The goal of these courses is to give students the information, abilities, and real-world experience they need to succeed in the data science industry. An outline of the typical content of a data science course is provided below, read the full info here:
- Foundational Concepts: Data science courses often start with foundational concepts in mathematics, statistics, and computer science. This may include topics such as linear algebra, calculus, probability theory, and programming languages like Python or R.
- Data Manipulation and Cleaning: Students learn techniques for acquiring, cleaning, and preprocessing raw data. This involves understanding different data formats, handling missing values, and dealing with outliers to ensure the quality and integrity of the data.
- Exploratory Data Analysis (EDA): EDA is a crucial step in the data science process, where students learn to visualize and summarize data to gain insights and identify patterns or trends. Techniques such as data visualization, descriptive statistics, and data summarization are covered in this part of the course.
- Statistical Analysis: Data science courses typically include a thorough grounding in statistical methods and techniques. Students learn how to apply statistical principles to analyze data, make inferences, and test hypotheses.
- Machine Learning: Machine learning forms a core component of data science courses. Students are introduced to various machine learning algorithms and techniques for supervised learning, unsupervised learning, and reinforcement learning. This includes regression, classification, clustering, dimensionality reduction, and ensemble methods.
- Big Data Technologies: With the increasing volume and complexity of data, knowledge of big data technologies becomes essential for data scientists. Courses may cover distributed computing frameworks like Hadoop and Spark, as well as tools and platforms for managing and processing large-scale data sets.
- Deep Learning and Neural Networks: Advanced data science courses may delve into deep learning, a subset of machine learning that focuses on neural networks and hierarchical representations of data. Students learn how to design, train, and evaluate deep learning models for tasks such as image recognition, natural language processing, and sequence prediction.
- Real-World Projects and Case Studies: Hands-on experience is a key component of data science courses. Students typically work on real-world projects or case studies, where they apply their knowledge and skills to solve practical problems. This allows them to gain valuable experience and develop a portfolio of work to showcase to potential employers.
- Ethical and Legal Considerations: Data science courses often address ethical and legal issues related to data privacy, security, bias, and fairness. Students learn about ethical guidelines, regulations, and best practices for responsible data handling and decision-making.
All things considered, the goal of data science courses is to give students a thorough understanding of the data science process, including data preparation and gathering as well as analysis, modelling, and interpretation. Through a blend of academic understanding and hands-on training, these courses equip students for professions in numerous fields where data is essential for creativity and decision-making.
What Is The Main Purpose Of Data Science?
Data science’s primary goal is to draw useful conclusions and information from massive, complicated databases to support decision-making, address issues, and spur creativity. The following are some significant facets of data science’s primary goal:
- Extracting Insights: Data science involves using various statistical, mathematical, and computational techniques to analyze data and uncover patterns, trends, and relationships that may not be immediately apparent. By extracting insights from data, organizations can gain a deeper understanding of their operations, customers, and markets.
- Predictive Modeling: Data science encompasses the development and application of predictive models to forecast future outcomes based on historical data. These models can be used for a wide range of purposes, such as predicting customer behaviour, forecasting sales demand, or identifying potential risks and opportunities.
- Optimization and Decision Support: Data science helps organizations optimize processes, resources, and strategies by leveraging data-driven insights. By identifying inefficiencies, bottlenecks, or areas for improvement, data science enables organizations to make more informed decisions and allocate resources more effectively.
- Personalization and Recommendation Systems: Data science plays a crucial role in enabling personalized experiences and recommendation systems in various domains, such as e-commerce, content streaming, and social media. By analyzing user data and preferences, organizations can tailor products, services, and content to individual users, enhancing customer satisfaction and engagement.
- Fraud Detection and Risk Management: Data science techniques are widely used for detecting anomalies, identifying fraudulent activities, and managing risks in industries such as finance, insurance, and cybersecurity. By analyzing patterns and anomalies in data, organizations can detect and mitigate potential threats and vulnerabilities.
- Healthcare and Life Sciences: In healthcare and life sciences, data science is used for various applications, including disease prediction, drug discovery, personalized medicine, and patient care optimization. By analyzing large volumes of clinical, genomic, and health-related data, data science can improve patient outcomes, accelerate medical research, and enhance healthcare delivery.
- Business Intelligence and Data-driven Decision Making: Data science enables organizations to transform raw data into actionable insights and intelligence that support strategic decision-making. By harnessing the power of data analytics, visualization, and reporting tools, organizations can gain a competitive edge, identify new opportunities, and mitigate risks.
In general, the primary goal of data science is to use data as a strategic asset, empowering businesses to effectively innovate, make better decisions, and accomplish their goals. Data science enables businesses to maximize the value of their data and promote long-term success by fusing subject knowledge, analytical abilities, and technology solutions.
Conclusion
With the main goal of gleaning knowledge and insights that may be put to use from large, complicated databases, data science is a field that is transforming. Organizations can find trends, forecast future results, streamline procedures, and make well-informed decisions by utilizing data science, which combines statistical, mathematical, and computational methods.
Organizations may improve healthcare, enhance experiences, identify fraud, and spur innovation across a range of industries by utilizing data. A culture of evidence-based decision-making is also promoted by data science, enabling people and organizations to use data as a strategic asset for development and success.
Data science will become increasingly important as we move through this data-driven era, influencing how we see the world, come to conclusions, and create. Data science is at the vanguard of technological innovation, influencing business, research, healthcare, and society at large. Its influence is exponential and will continue to shape the future.